Note
Go to the end to download the full example code.
Machine-learning vs. Information theoretic approaches for HOI#
This example compares Machine-learning and Information theoretic approaches to investigate Higher Order Interactions.
import numpy as np
from sklearn.discriminant_analysis import LinearDiscriminantAnalysis
from sklearn.model_selection import cross_val_score
from hoi.metrics import GradientOinfo
import matplotlib.pyplot as plt
plt.style.use('ggplot')
Data simulation#
Let’s start by creating a function that simulates higher-order interactions between a multivariate variable \(X={X_{1}, ..., X_{N}}\) and a univariate variable \(Y\). We are then going to create redundant and synergistic relationships between \(X\) and \(Y\). To introduce redundancy between the two, each \(X_{i}\) is going to receive a copy of \(Y\). To create synergy, each \(X_{i}\) is going to encode different parts of \(Y\) so that \(Y\) can only be fully known when all the \(X_{i}\) are provided. For further information about how to simulate redundant and synergistic interactions, checkout the example How to simulate redundancy and synergy
def simulate_data(
n_samples=300,
n_x=3,
n_times=100,
t_start=40,
t_end=60,
rel_type="redundancy",
rel_strength=0.2,
):
"""Data simulation.
Parameters
----------
n_samples : int, 300
Number of samples in x and y
n_x : int, 3
Number of features in x
n_times : int, 100
Number of time points in x
t_start : int, 40
Time sample at which the relation between x and y starts
t_end : int, 60
Time sample at which the relation between x and y ends
rel_type : {"redundancy", "synergy"}
Specify whether the nature of the relationship between x and y. Use
either "redundancy" or "synergy"
rel_strength : float, 0.2
Strength of the statistical dependency between x and y
Returns
-------
x : array_like
Array of shape (n_samples, n_x, n_times)
y : array_like
Target array of shape (n_samples)
"""
assert rel_type in ["redundancy", "synergy"]
sl = slice(t_start, t_end)
hann = np.hanning(t_end - t_start)
y = np.random.permutation([0] * n_samples + [1] * n_samples)
y_norm = 2 * y - 1
if rel_type == "redundancy":
x = np.random.rand(2 * n_samples, n_x, n_times)
x[..., sl] += (
rel_strength * y_norm.reshape(-1, 1, 1) * hann.reshape(1, 1, -1)
)
elif rel_type == "synergy":
x = np.random.rand(2 * n_samples, n_x, n_times)
trial_blocks = np.array_split(np.arange(n_samples * 2), n_x)
for n_r in range(n_x):
_trials = trial_blocks[n_r]
x[_trials, n_r, sl] += (
rel_strength
* y_norm[_trials].reshape(-1, 1)
* hann.reshape(1, -1)
)
return x, y
Now we can create two pairs of variables \((X_{red}, Y_{red})\) and \((X_{syn}, Y_{syn})\) with respectively redundant and synergistic relationships between them
x_red, y_red = simulate_data(rel_type="redundancy", rel_strength=0.2)
x_syn, y_syn = simulate_data(rel_type="synergy", rel_strength=0.7)
Let’s plot the data
def plot_xy(x, y):
for n_y, u in enumerate(np.unique(y)):
for n_x in range(x.shape[1]):
u_x = x[y == u, n_x, :]
x_m = u_x.mean(0)
plt.plot(x_m, color=f"C{n_y}", label=rf"$X[Y == {u}, {n_x}, :]$")
plt.legend()
fig, axs = plt.subplots(2, 1, figsize=(8, 12), sharex=True, sharey=True)
axs = np.ravel(axs)
plt.sca(axs[0])
plot_xy(x_red, y_red)
plt.title("Redundant interactions")
plt.sca(axs[1])
plot_xy(x_syn, y_syn)
plt.title("Synergistic interactions")
plt.show()
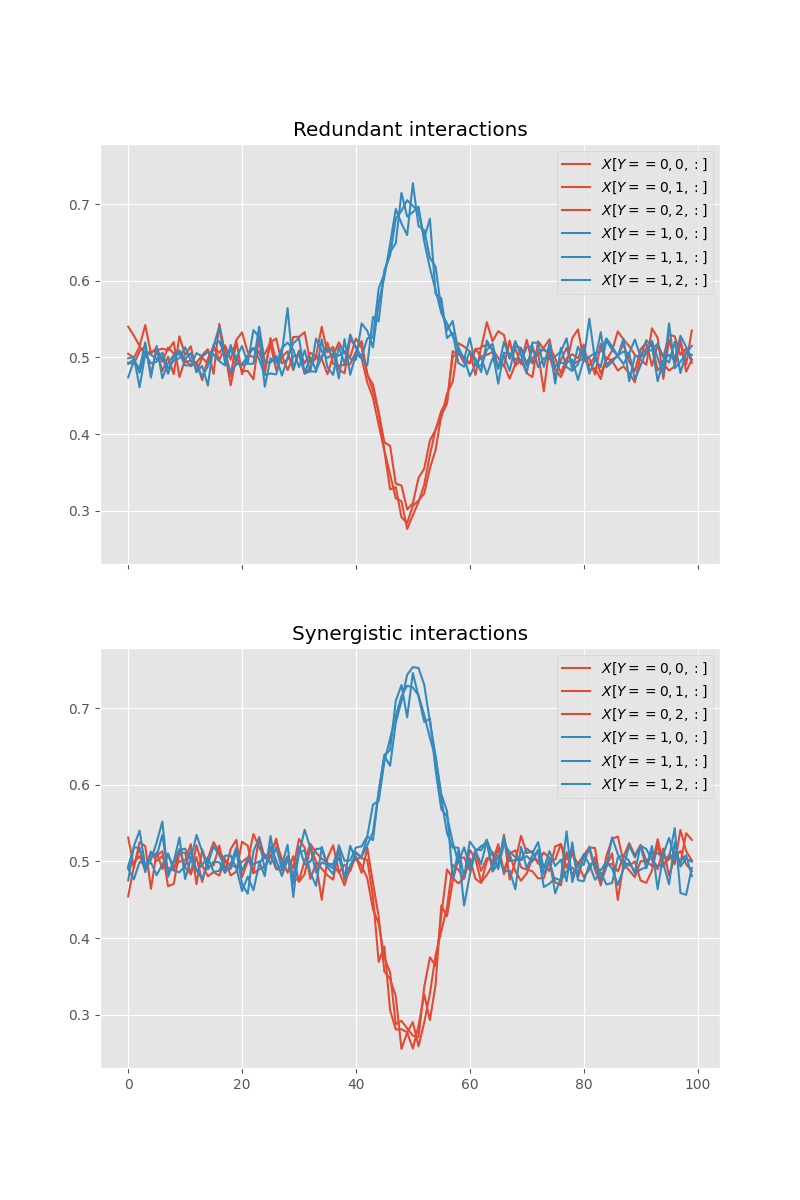
Decoding Y using X#
Now let’s try to decode the \(Y\) variable using \(X\).
def decode_y(x, y):
clf = LinearDiscriminantAnalysis()
_, n_x, n_times = x.shape
da = np.zeros((n_x + 1, n_times))
for t in range(n_times):
for n_r in range(n_x):
da[n_r, t] = cross_val_score(clf, x[:, n_r, [t]], y, cv=5).mean()
da[-1, t] = cross_val_score(clf, x[:, :, t], y, cv=5).mean()
return 100 * da
def plot_decoding(da):
for k in range(da.shape[0] - 1):
plt.plot(da[k, :], color="k", label=r"$DA_{X_{%i}}$" % (k + 1))
plt.plot(da[-1, :], color="C0", label=r"$DA_{X_{1}, ..., X_{N}}$", lw=4)
plt.legend()
fig, axs = plt.subplots(2, 1, figsize=(8, 12), sharex=True, sharey=True)
axs = np.ravel(axs)
plt.sca(axs[0])
plot_decoding(decode_y(x_red, y_red))
plt.ylabel("Decoding accuracy [%]")
plt.title("Redundant interactions", fontweight="bold")
plt.sca(axs[1])
plot_decoding(decode_y(x_syn, y_syn))
plt.ylabel("Decoding accuracy [%]")
plt.title("Synergistic interactions", fontweight="bold")
plt.show()
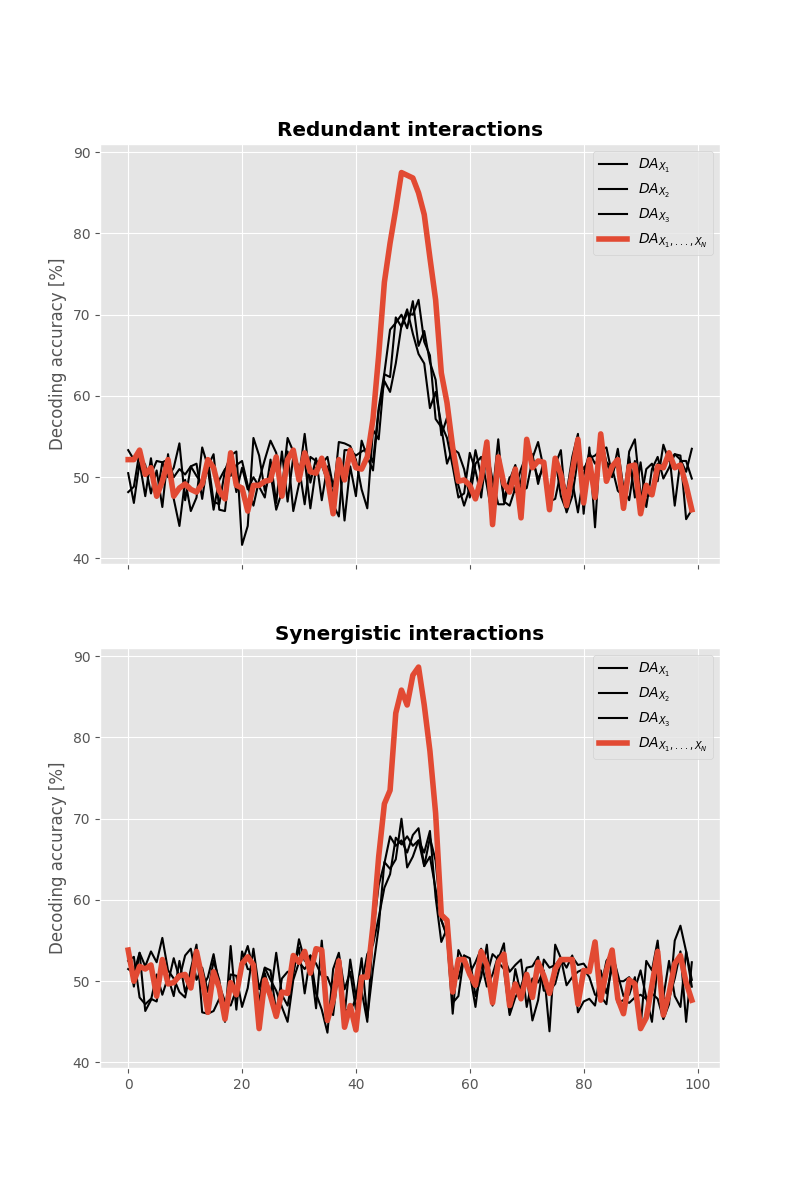
As we can see, using machine-learning we can decode the \(Y\) variable with a decoding accuracy of ~90% when there’s either redundant or synergistic interactions between \(X\) and \(Y\)
Using information-theoretic approaches#
Now let’s information-theoretic approaches. The question we try to answer here is whether the \(X_{i}\) are carrying redundant or synergistic information about \(Y\). To answer this question we are going to use the Gradient Oinfo.
def it(x, y):
model = GradientOinfo(x, y, verbose=False)
return model.fit(minsize=3, maxsize=3)
fig, axs = plt.subplots(2, 1, figsize=(8, 12), sharex=True, sharey=True)
axs = np.ravel(axs)
plt.sca(axs[0])
plt.plot(it(x_red, y_red).squeeze())
plt.ylabel(r"$\partial \Omega_{[X_{1}, ..., X_{N}]}$ [Bits]")
plt.title("Redundant interactions", fontweight="bold")
plt.sca(axs[1])
plt.plot(it(x_syn, y_syn).squeeze())
plt.ylabel(r"$\partial \Omega_{[X_{1}, ..., X_{N}]}$ [Bits]")
plt.title("Synergistic interactions", fontweight="bold")
plt.show()
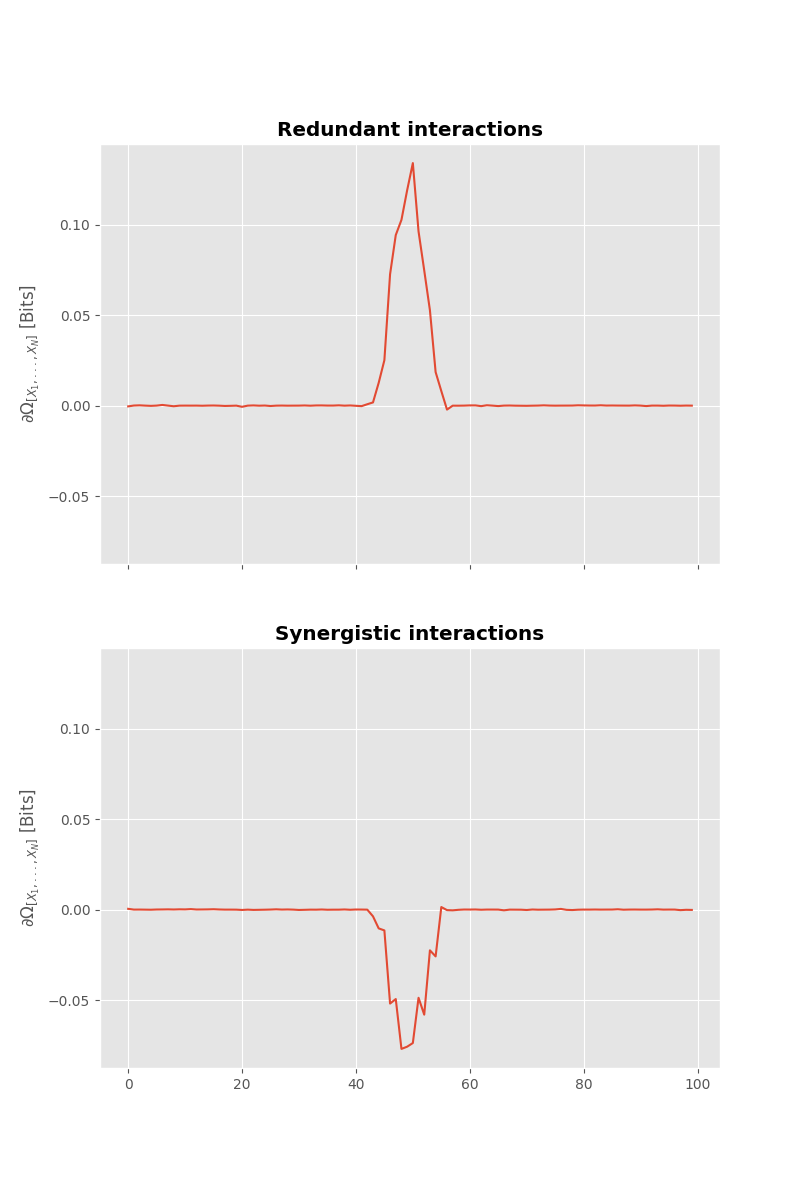
We retrieve the bump of information around sample 50 however this time, the bump is positive in case of redundant interactions and negative in case of synergistic interactions.
Total running time of the script: (0 minutes 9.275 seconds)