Note
Go to the end to download the full example code
Compare within-subjects statistics when computing mutual information#
This example illustrates how to define and run a workflow for computing mutual information and evaluate stastitics. Inference are made within-subjects (fixed effect = ffx).
import numpy as np
from frites.simulations import sim_multi_suj_ephy, sim_mi_cc
from frites.dataset import DatasetEphy
from frites.workflow import WfMi
from frites import set_mpl_style
import matplotlib.pyplot as plt
set_mpl_style()
Simulate electrophysiological data#
Let’s start by simulating MEG / EEG electrophysiological data coming from
multiple subjects using the function
frites.simulations.sim_multi_suj_ephy()
. As a result, the data output
is a list of length n_subjects of arrays, each one with a shape of
n_epochs, n_sites, n_times
modality = 'meeg'
n_subjects = 5
n_epochs = 400
n_times = 100
data, roi, time = sim_multi_suj_ephy(n_subjects=n_subjects, n_epochs=n_epochs,
n_times=n_times, modality=modality,
random_state=0)
Simulate mutual information#
Once the data have been created, we simulate an increase of mutual
information by creating a continuous variable y using the function
frites.simulations.sim_mi_cc()
. This allows to simulate model-based
analysis by computing $I(data; y)$ where data and y are two continuous
variables
y, _ = sim_mi_cc(data, snr=.1)
Create an electrophysiological dataset#
Now, we use the frites.dataset.DatasetEphy
in order to create a
compatible electrophysiological dataset
dt = DatasetEphy(data, y=y, roi=roi, times=time, verbose=False)
Define the workflow#
We now define the workflow for computing mi and evaluate statistics using the
class frites.workflow.WfMi
. Here, the type of mutual
information to perform is ‘cc’ between it’s computed between two continuous
variables. And we also specify the inference type ‘ffx’ for fixed-effect
Compute the mutual information and statistics#
# list of corrections for multiple comparison
mcps = ['cluster', 'maxstat', 'fdr', 'bonferroni']
kw = dict(n_jobs=1, n_perm=200)
"""
The `cluster_th` input parameter specifies how the threshold is defined.
Use either :
* a float for a manual threshold
* None and it will be infered using the distribution of permutations
* 'tfce' for a TFCE threshold
"""
cluster_th = 'tfce' # {float, None, 'tfce'}
plt.figure(figsize=(10, 8))
for mcp in mcps:
print(f'-> FFX / MCP={mcp}')
mi, pvalues = wf.fit(dt, mcp=mcp, cluster_th=cluster_th, **kw)
# remove p-values that exceed 0.05
pvalues.data[pvalues >= .05] = 1.
plt.subplot(212)
plt.plot(time, pvalues.squeeze(), label=f'ffx-{mcp}')
plt.xlabel('Time (s)'), plt.ylabel("P-values")
plt.title("P-values (ffx)")
plt.legend()
plt.subplot(211)
plt.plot(time, mi.squeeze())
plt.ylabel("Bits")
plt.title("Mutual information I(C; C)")
plt.show()
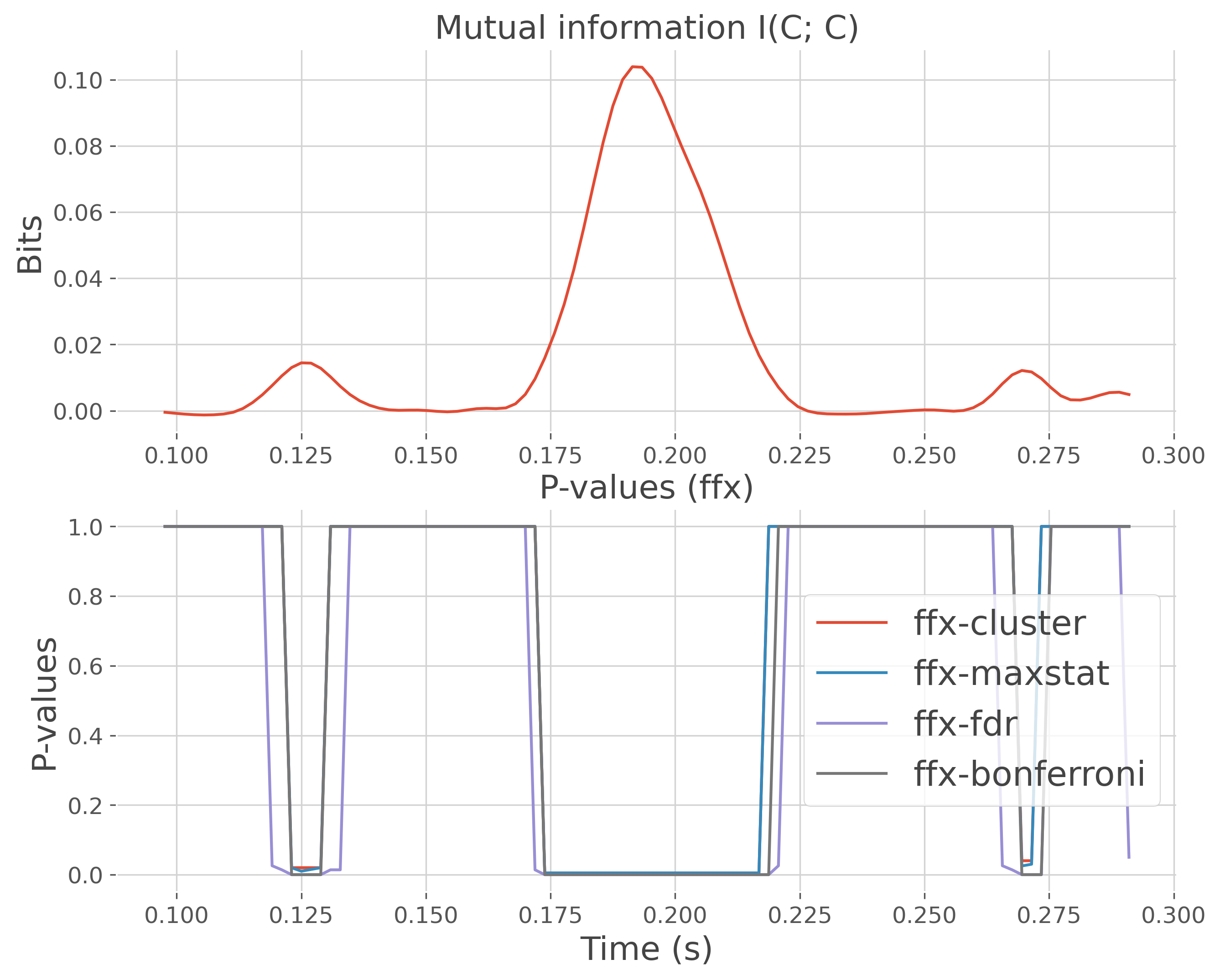
-> FFX / MCP=cluster
-> FFX / MCP=maxstat
-> FFX / MCP=fdr
-> FFX / MCP=bonferroni
Total running time of the script: (0 minutes 2.896 seconds)
Estimated memory usage: 9 MB